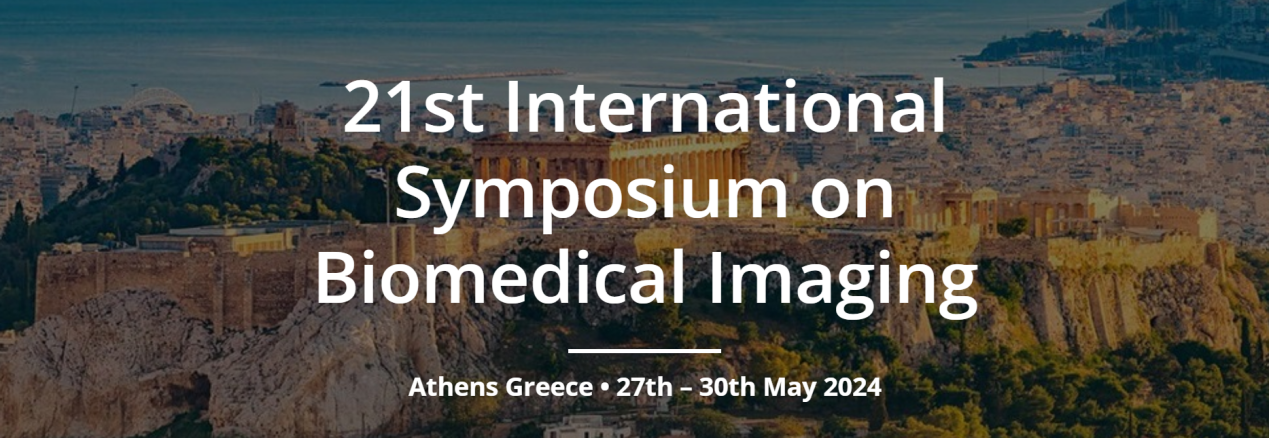
The 2024 IEEE International Symposium on Biomedical Imaging (ISBI 2024) was held from May 27 to May 30, 2024, in Athens, Greece. The conference was co-hosted by the IEEE Signal Processing Society (SPS) and the Engineering in Medicine and Biology Society (EMBS). IEEE ISBI is one of the top scientific conferences covering mathematical, algorithmic, and computational aspects of biomedical imaging, focusing on topics such as artificial intelligence, computer vision, and pattern recognition.
This year, Medical Imaging, Robotics, Analytic Computing Laboratory/Engineering (MIRACLE) Lab had six papers accepted, with five of them being invited for oral presentations. Sun Dai, a 2023 PhD student from the School of Biomedical Engineering at USTC, was the first author of DNA-DIR, which was nominated for the Best Oral Award. Gao Ziqi, a 2022 master’s student, was awarded the Student Travel Grant.
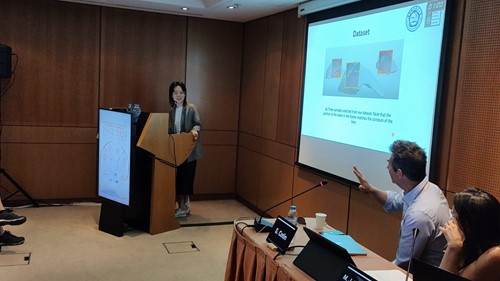
Research Achievement 1:
1. DNA-DIR: 2D-3D Geometry Embedding for Intraoperative Partial-to-Full Registration
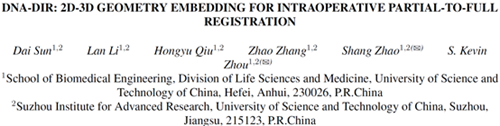
This paper introduces a novel progressive registration pipeline, DNA-DIR, which addresses the non-rigid alignment between partial and full 2D-3D images in robot-assisted surgery (RAS) for liver procedures with robust posture requirements. Additionally, the paper provides a simulated 2D-3D registration dataset, including 150 accurately annotated cases from patients with different liver diseases.
Research Achievement 2:
2) CMUNeXt: An Efficient Medical Image Segmentation Network Based on Large Kernel and Skip Fusion
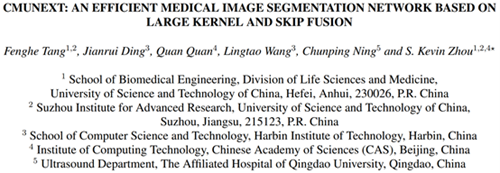
This paper proposes an efficient and lightweight segmentation network, CMUNeXt, which employs large convolutional kernels and an anti-bottleneck design to fully mix long-range spatial and positional information, effectively extracting global contextual information. The Skip-fusion module is introduced to enable smooth skip connections and ensure adequate feature fusion.
Research Achievement 3:
3) Rethinking Dual-Domain Undersampled MRI Reconstruction: Domain-Specific Design from the Perspective of the Receptive Field
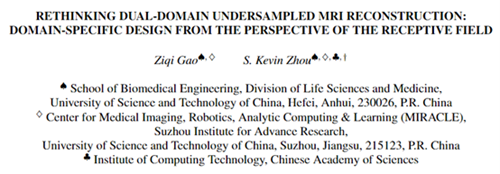
This paper presents a dual-domain under sampled MRI reconstruction network that incorporates domain-specific designs based on the characteristics of the image and frequency domains. The design is inspired by the receptive field of neural networks to address the task of under sampled MRI reconstruction.
Research Achievement 4:
4) Nowhere to Hide: Toward Robust Reactive Medical Adversarial Defense
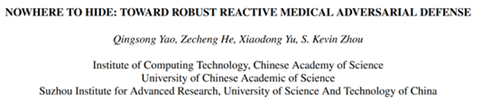
This paper introduces an adversarial attack detector called the Medical Adversarial Shield, which uses adversarial training to capture traces of adversarial noise in cascade features, especially in shallow layers. It achieves over 90% detection accuracy against the strongest adaptive white-box and common attacks.
Research Achievement 5:
5) Long-Tailed Multi-Label Classification with Noisy Labels of Thoracic Diseases from Chest X-rays
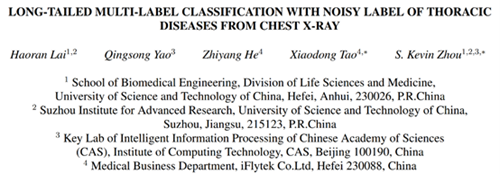
This study builds a new long-tailed multi-label dataset called “LTML-MIMIC-CXR,” covering 39 diseases, including 26 newly added rare diseases. A new benchmark method is proposed, using adaptive negative regularization and a large-loss reconsideration strategy to address the issues of over-suppressed negative logits in long-tailed classes and noisy labels generated by automated annotation.
Research Achievement 6:
6) E-DM: Evaluating Diffusion Model by Conformal Prediction
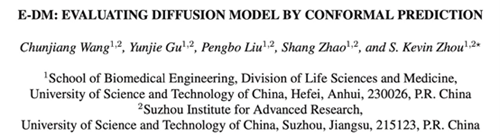
Diffusion models have gained widespread attention for their exceptional performance in multiple fields. However, evaluating the reliability and quality of images generated by these models remains a challenge. This paper introduces conformal prediction as a method for uncertainty quantification, termed E-DM, aimed at providing uncertainty control without compromising image quality.
(School of Biomedical Engineering)