The Jiushao team at the University of Science and Technology of China (USTC) has made significant progress in studying the fundamental mechanisms behind mode collapse. Through mathematical analysis, they proposed a new method to quantitatively detect and solve the mode collapse issue in Generative Adversarial Networks (GANs). This research, titled “DynGAN: Solving Mode Collapse in GANs with Dynamic Clustering,” has been published in the internationally renowned journal IEEE Transactions on Pattern Analysis and Machine Intelligence.
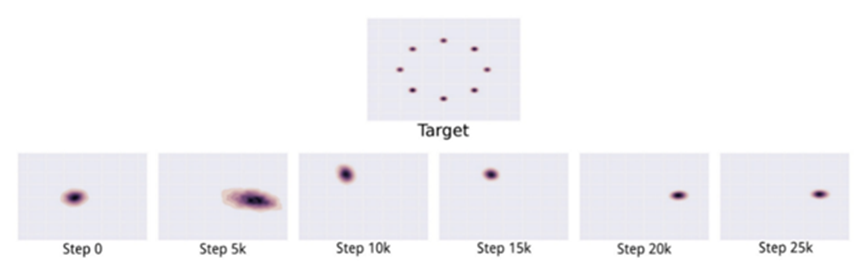
Figure 1: What is Mode Collapse?
(Note: Figure excerpted from the literature: Goodfellow, Ian. “NIPS 2016 tutorial: Generative adversarial networks.” arXiv preprint arXiv:1701.00160 (2016).)
Generative Adversarial Networks (GANs) are widely used generative models that learn the distribution of real samples to synthesize complex and realistic data. However, mode collapse is a significant challenge in GANs, where the diversity of the generated samples is noticeably lower than the diversity of the real samples. This poses a problem for further applications. Due to the complexity of GANs and their training process, which involves multiple potential factors and stages, the exact mechanism that leads to mode collapse has long remained unclear.
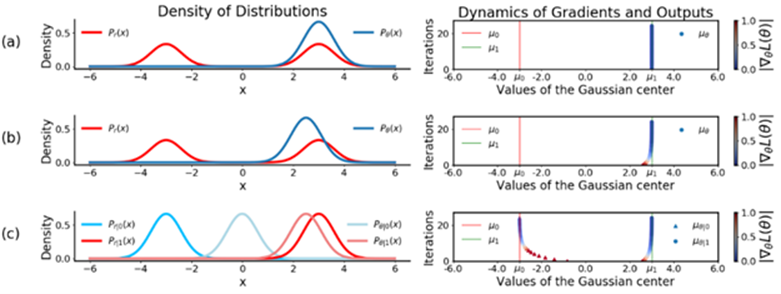
Figure 2: The Fundamental Mechanism Behind Mode Collapse
Through in-depth research, the team discovered the fundamental mechanism that causes mode collapse in GANs. Theoretical analysis revealed that when real data consists of multiple modes, the generator's loss function is non-convex with respect to its parameters. Specifically, the parameters that lead to the generated distribution covering only part of the real distribution's modes are local minima of the generator's loss function.


Figure 3: A New Method to Solve Mode Collapse
To address the mode collapse issue, the team proposed a unified framework called Dynamic GAN (DynGAN). This method sets appropriate thresholds for the observable discriminator output to detect the samples that the generator fails to generate (collapsed samples). The training set is then partitioned based on these collapsed samples, and dynamic conditional generative models are trained on these partitions.
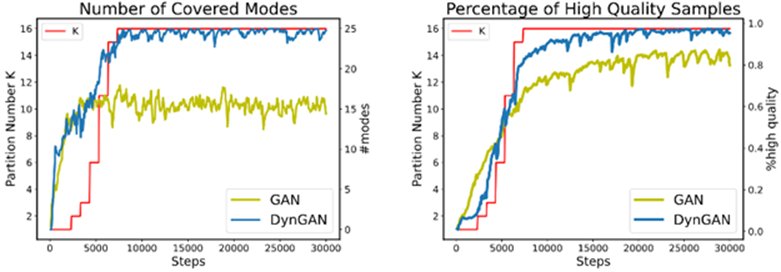
Figure 4: Performance of DynGAN on Synthetic Datasets
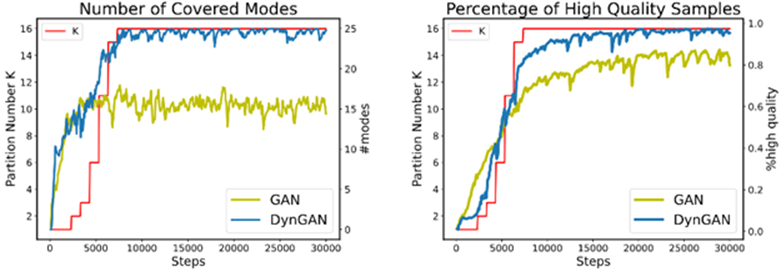
Figure 5: Effectiveness of DynGAN on Real-World Datasets
The theoretical results guarantee that the proposed DynGAN method achieves progressive mode coverage. Experiments on both synthetic and real-world datasets show that DynGAN outperforms existing GANs and their variants in overcoming mode collapse. This research not only advances the theoretical understanding of GANs but also provides a practical solution to improve mode coverage in generative models.
Dr. Yixin Luo and Prof. Zhouwang Yang from USTC are the first author and corresponding author of the work, respectively. This research was supported by major research programs of the National Natural Science Foundation of China, the Ministry of Science and Technology's Key Research Program on “Mathematics and Applied Research,” the Chinese Academy of Sciences' Strategic Priority Research Program, and the Major Scientific and Technological Projects of Anhui Province.
Paper link: https://doi.org/10.1109/TPAMI.2024.3367532
Yixin Luo, Zhouwang Yang. DynGAN: Solving Mode Collapse in GANs with Dynamic Clustering. IEEE Transactions on Pattern Analysis and Machine Intelligence, p1-12, 20 February 2024.*
(Department of Mathematics, Research Office)
Transferred from: USTC Official Website
Dr. Yixin Luo is currently a postdoctoral researcher at the Suzhou Institute for Advanced Research, University of Science and Technology of China.